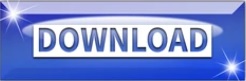
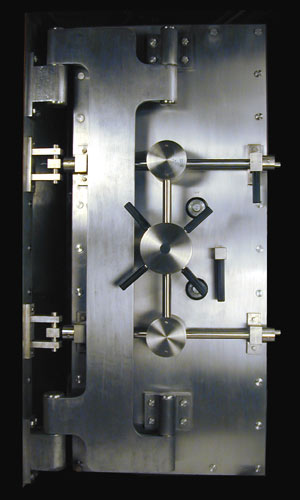
If data are not conforming to these definitions and rules then that is a problem for the business, not the data warehouse. The design is flexible, scalable, consistent and adaptable to the needs of the enterprise" ĭata vault's philosophy is that all data is relevant data, even if it is not in line with established definitions and business rules. It is a hybrid approach encompassing the best of breed between 3rd normal form (3NF) and star schema. "The Data Vault Model is a detail oriented, historical tracking and uniquely linked set of normalized tables that support one or more functional areas of business. Data vault is designed to avoid or minimize the impact of those issues, by moving them to areas of the data warehouse that are outside the historical storage area (cleansing is done in the data marts) and by separating the structural items (business keys and the associations between the business keys) from the descriptive attributes.ĭan Linstedt, the creator of the method, describes the resulting database as follows: For conformed dimensions you also have to cleanse data (to conform it) and this is undesirable in a number of cases since this inevitably will lose information. Both techniques have issues when dealing with changes in the systems feeding the data warehouse. Either you model according to Ralph Kimball, with conformed dimensions and an enterprise data bus, or you model according to Bill Inmon with the database normalized. In data warehouse modeling there are two well-known competing options for modeling the layer where the data are stored. ( August 2019) ( Learn how and when to remove this template message)
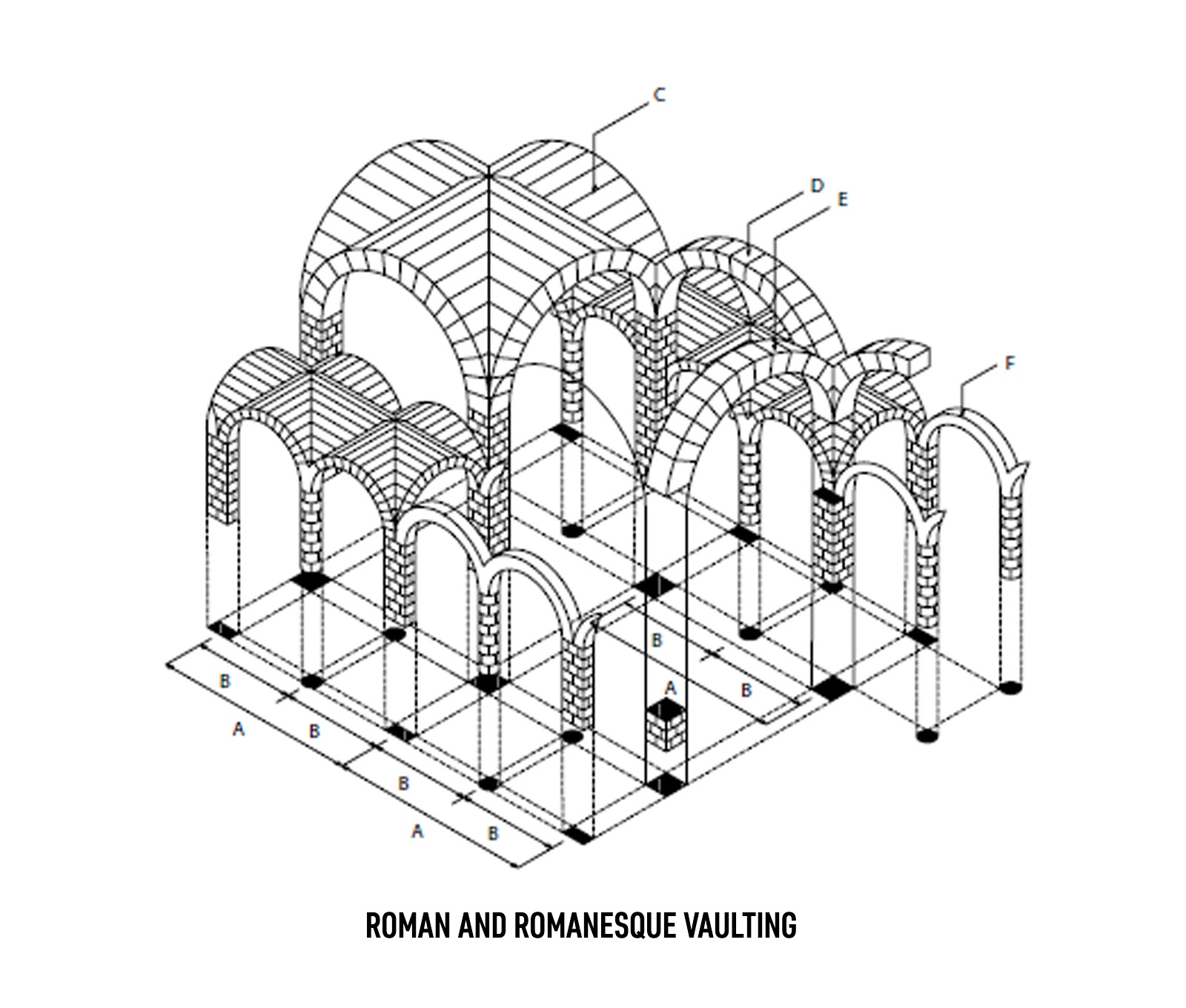
Statements consisting only of original research should be removed. Please improve it by verifying the claims made and adding inline citations. Relevant discussion may be found on Template talk:Original research. This article possibly contains original research. Both data vaults and anchor models are entity-based models, but anchor models have a more normalized approach. Unlike the star schema ( dimensional modelling) and the classical relational model (3NF), data vault and anchor modelling are well-suited for capturing changes that occur when a source system is changed or added, but are considered advanced techniques which require experienced data architects. Data vault is designed to enable parallel loading as much as possible, so that very large implementations can scale out without the need for major redesign. The modeling method is designed to be resilient to change in the business environment where the data being stored is coming from, by explicitly separating structural information from descriptive attributes. A data vault enterprise data warehouse provides both a single version of facts and a single source of truth. This is summarized in the statement that a data vault stores " a single version of the facts" (also expressed by Dan Linstedt as "all the data, all of the time") as opposed to the practice in other data warehouse methods of storing "a single version of the truth" where data that does not conform to the definitions is removed or "cleansed". The concept was published in 2000 by Dan Linstedt.ĭata vault modeling makes no distinction between good and bad data ("bad" meaning not conforming to business rules). This means that every row in a data vault must be accompanied by record source and load date attributes, enabling an auditor to trace values back to the source. It is also a method of looking at historical data that deals with issues such as auditing, tracing of data, loading speed and resilience to change as well as emphasizing the need to trace where all the data in the database came from. Simple data vault model with two hubs (blue), one link (green) and four satellites (yellow)ĭata vault modeling, also known as common foundational warehouse architecture or common foundational modeling architecture, is a database modeling method that is designed to provide long-term historical storage of data coming in from multiple operational systems.
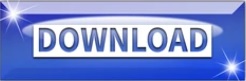